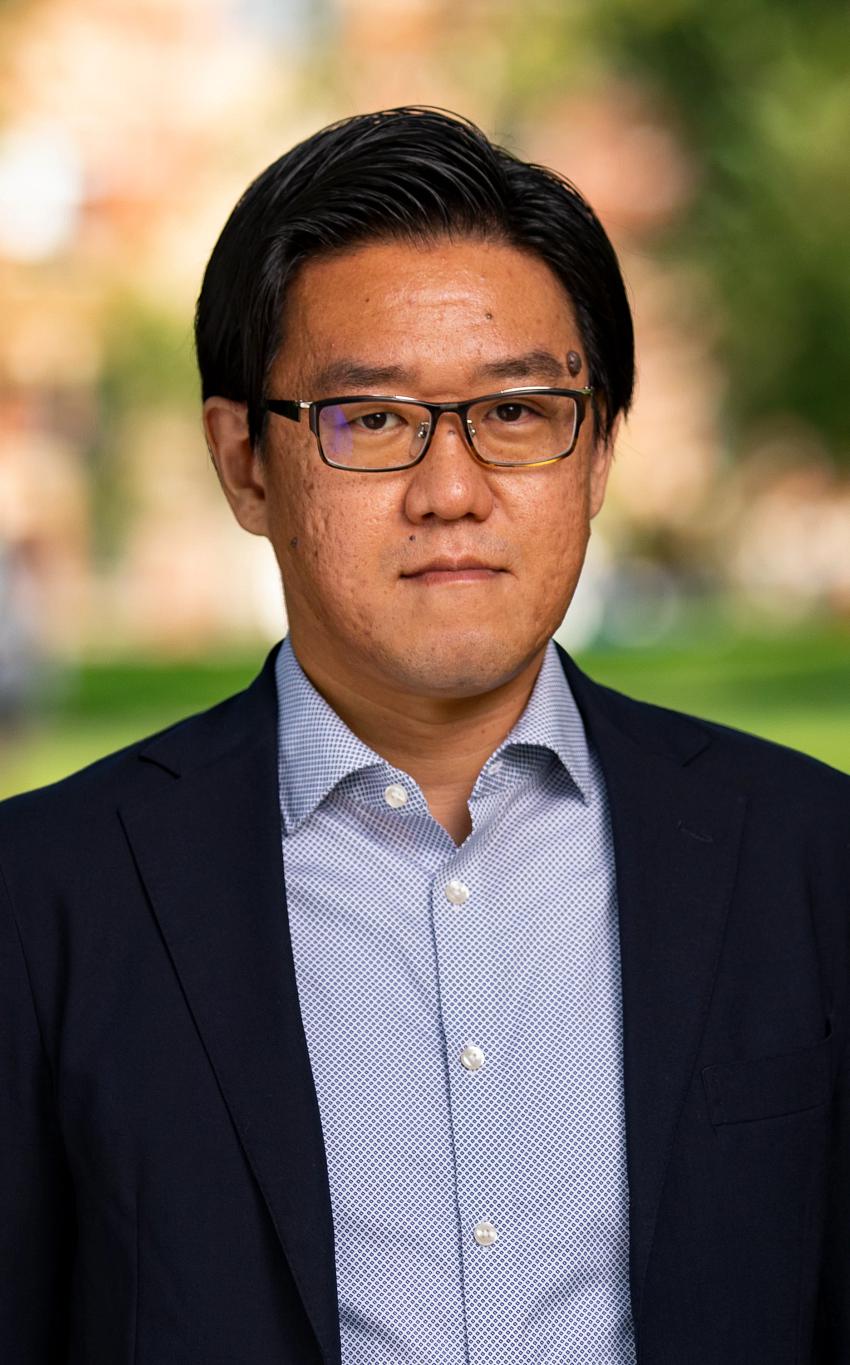 |
Date |
December/8/2022 |
Time |
Japan Standard Time (UTC+9h) |
|
9:00~10:30 |
Speaker |
Toru Kitagawa Brown University and University College London
|
Title |
Policy Choice in Time Series by Empirical Welfare Maximization
|
Abstract |
This paper develops a novel method for policy choice in a dynamic setting where the available data is a multivariate time series. Building on the statistical treatment choice framework, we propose Time-series Empirical Welfare Maximization (T-EWM) methods to estimate an optimal policy rule for the current period or over multiple periods by maximizing an empirical welfare criterion constructed using nonparametric potential outcome time-series. We characterize conditions under which T-EWM consistently learns a policy choice that is optimal in terms of conditional welfare given the time-series history. We then derive a non-asymptotic upper bound for conditional welfare regret and its minimax lower bound. To illustrate the implementation and uses of T-EWM, we perform simulation studies and apply the method to estimate optimal monetary policy rules from macroeconomic time-series data.
|
Video |
Click here to watch the video
|
Chair |
Ryo Okui The University of Tokyo
|
Hosts |
TEDS, JSPS KAKENHI (B) No. 22H00833 (Liu).
|
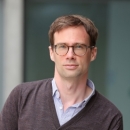 |
Date |
November/24/2022 |
Time |
Japan Standard Time (UTC+9h) |
|
17:00~18:30 |
Speaker |
Koen Jochmans Toulouse School of Economics
|
Title |
Bootstrap inference for fixed-effect models
|
Abstract |
The maximum-likelihood estimator of nonlinear panel data models with fixed effects is asymptotically biased under rectangular-array asymptotics. The literature has devoted substantial effort to devising methods that correct for this bias as to salvage standard inferential procedures. The chief purpose of this paper is to show that the (recursive, parametric) bootstrap replicates the distribution of the (uncorrected) maximum-likelihood estimator in large samples. This justifies the use of confidence sets constructed via conventional bootstrap methods. No adjustment for the presence of bias needs to be made.
|
Video |
Click here to watch the video
|
Chair |
Ryo Okui The University of Tokyo
|
Hosts |
TEDS, JSPS KAKENHI (B) No. 22H00833 (Liu).
|
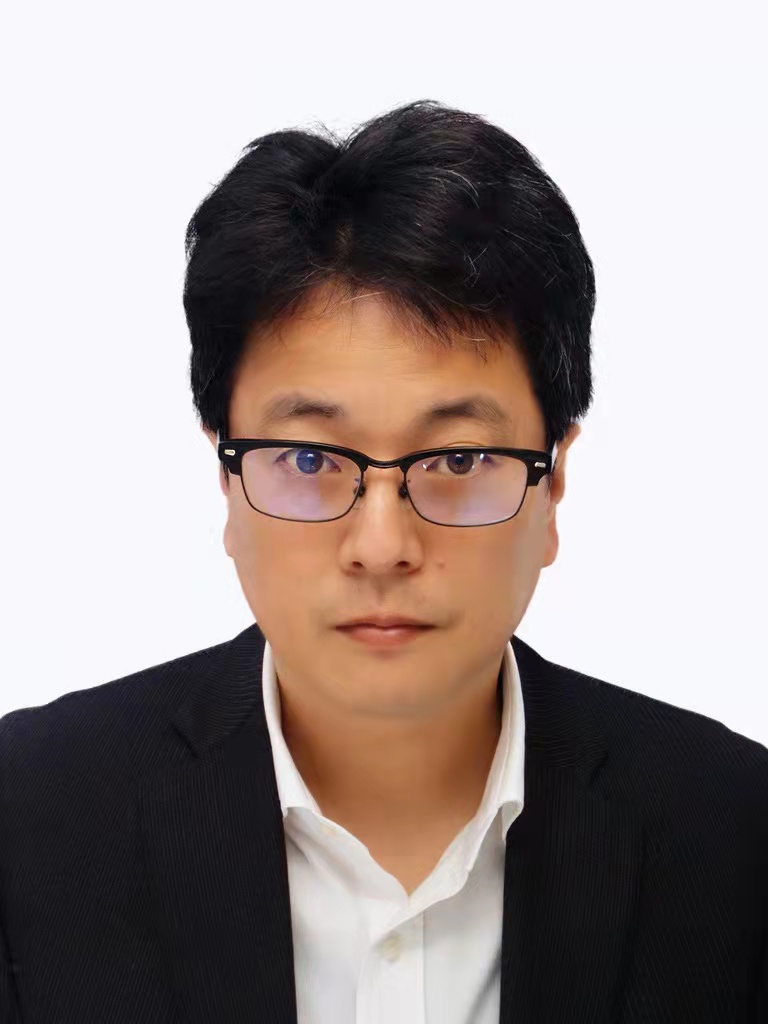 |
Date |
November/8/2022 |
Time |
Japan Standard Time (UTC+9h) |
|
10:00~11:30 |
Speaker |
Yohei Yamamoto Hitotsubashi University
|
Title |
Anthropogenic influence on extremes and risk hotspots
|
Abstract |
Study of the frequency and magnitude of climate extremes as the world warms is of utmost importance, especially separating the influence of natural and anthropogenic forcing factors. Record-breaking temperature and precipitation events were studied using event-attribution techniques. Here, we provide spatial and temporal observation-based analyses of the role of natural and anthropogenic factors, using state-of-the-art time series methods. We show that the risk from extreme temperature and rainfall events has severely increased for most regions worldwide. We also identify risk hotspots defined as regions for which increased risk of extreme events and high exposure in terms of either high Gross Domestic Product (GDP) or large population are both present. For the year 2018, increased anthropogenic forcings are mostly responsible for increased risk to extreme temperature/precipitation affecting 94%/72% of global population and 97%/76% of global GDP relative to the baseline period 1961-1990.
|
Video |
Click here to watch the video
|
Chair |
Wenjie Wang Nanyang Technological University
|
Hosts |
TEDS and Nanyang Technological University
|
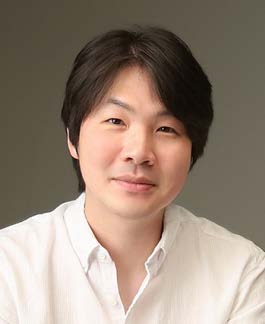 |
Date |
October/14/2022 |
Time |
Japan Standard Time (UTC+9h) |
|
10:00 ~ 11:30 |
Speaker |
Jungbin Hwang University of Connecticut
|
Title |
Fixed-Cluster Inference with Unbalanced Cluster Sizes
|
Abstract |
This paper provides asymptotic distribution and approximation theory for clustered data with a fixed number of large-sized clusters, allowing cluster sizes to be heterogeneous. In developing new inference procedures under fixed-cluster asymptotics, we make the following contributions. First, we show that the fixed-cluster asymptotic distribution of cluster-robust Wald statistic can be represented by a quadratic form of a standard normal vector with an
independent random weighting matrix, where the random weighting matrix is equal in distribution to a weighted sum of independent Wishart matrices. Second, we develop a convenient F-approximation to the nonstandard fixed-cluster limiting distribution. The key intuition is that a scaled Wishart matrix can well approximate the random weighting matrix in the fixed-cluster limit of the Wald statistic with a proper choice of degree of freedom. We also show that the equivalent degree of freedom in our F-approximation connects to the equivalent number of clusters in Carter et al.’s (2013). Lastly, we establish the fixed-G asymptotic distribution and F-approximation for the test based on the jackknife CCE and prove its built-in bias adjustment function.
|
Venue |
Click here to join the Zoom
Meeting Meeting ID:869 1606 0736
Passcode:104168
|
Chair |
Wenjie Wang Nanyang Technological University
|
Hosts |
TEDS and Nanyang Technological University
|
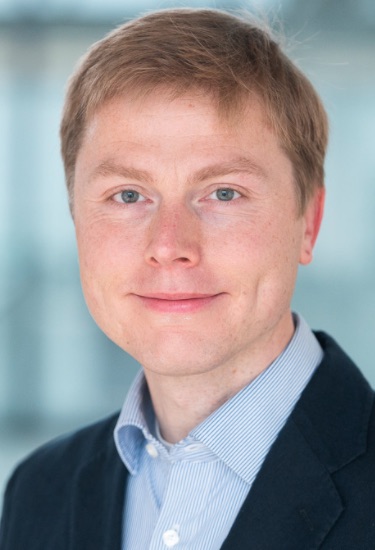 |
Date |
September/29/2022 |
Time |
Japan Standard Time (UTC+9h) |
|
17:00~18:30 |
Speaker |
Martin Weidner University of Oxford
|
Title |
Bounds on Average Effects in Discrete Choice Panel Data
|
Abstract |
Average effects in discrete choice panel data models with individual-specific fixed effects are generally only partially identified in short panels. While consistent estimation of the identified set is possible, it generally requires very large sample sizes, especially when the number of support points of the observed covariates is large, such as when the covariates are continuous. In this paper, we propose estimating outer bounds on the identified set of average effects. Our bounds are easy to construct, converge at the parametric rate, and are computationally simple to obtain even in moderately large samples, independent of whether the covariates are discrete or continuous. We also provide asymptotically valid confidence intervals on the identified set. Simulation studies confirm that our approach works well and is informative in finite samples. We also consider an application to labor force participation.
|
Video |
Click here to watch the video
|
Chair |
Ryo Okui The University of Tokyo
|
Hosts |
TEDS, JSPS KAKENHI (B) No. 22H00833 (Liu).
|
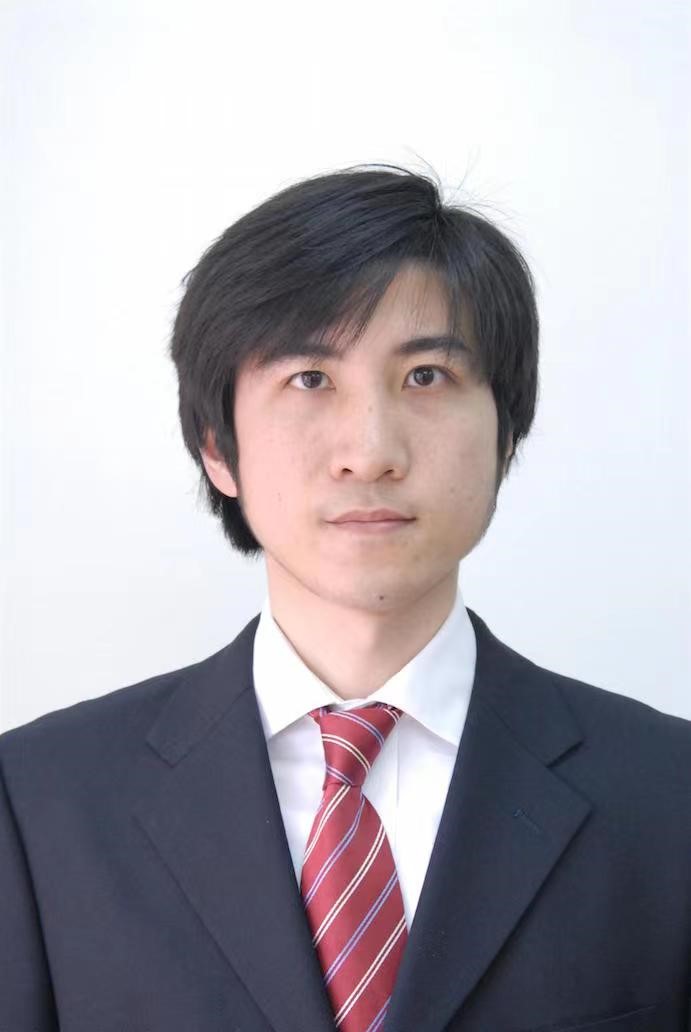 |
Date |
September/28/2022 |
Time |
Japan Standard Time (UTC+9h) |
|
10:00 |
Speaker |
Wenjie Wang Nanyang Technological University
|
Title |
Wild Bootstrap Inference for Instrumental Variables Regressions with Weak and Few Clusters
|
Abstract |
We study the wild bootstrap inference for instrumental variable regressions with a small number of large clusters. We first show that the wild bootstrap Wald test controls size asymptotically up to a small error as long as the parameters of endogenous variables are strongly identified in at least one of the clusters and has power against local alternatives if the parameters of endogenous variables are strongly identified in five or six clusters. We further develop a wild bootstrap Anderson-Rubin test for the full-vector inference and show that it controls size asymptotically even under weak identification in all clusters. We illustrate their good performance using simulations and provide an empirical application to a well-known dataset about US local labor markets.
|
Venue |
Click here to join the Zoom
Meeting Meeting ID: 9625852673
|
Chair |
Dayu Liu Jilin University
|
Hosts |
TEDS and Center for Quantitative Economics of Jilin University
|
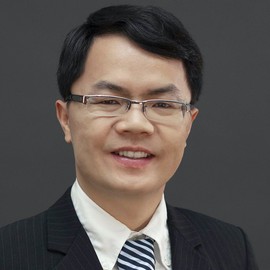 |
Date |
August/27/2022 |
Time |
Japan Standard Time (UTC+9h) |
|
9:30 |
Speaker |
Yang Feng New York University
|
Title |
Transfer Learning under High-dimensional Generalized Linear Models
|
Abstract |
In this work, we study the transfer learning problem under high-dimensional generalized linear models (GLMs), which aim to improve the fit on target data by borrowing information from useful source data. Given which sources to transfer, we propose a transfer learning algorithm on GLM, and derive its ℓ1/ℓ2-estimation error bounds as well as a bound for a prediction error measure. The theoretical analysis shows that when the target and source are sufficiently close to each other, these bounds could be improved over those of the classical penalized estimator using only target data under mild conditions. When we don't know which sources to transfer, an algorithm-free transferable source detection approach is introduced to detect informative sources. The detection consistency is proved under the high-dimensional GLM transfer learning setting. We also propose an algorithm to construct confidence intervals of each coefficient component, and the corresponding theories are provided. Extensive simulations and a real-data experiment verify the effectiveness of our algorithms. We implement the proposed GLM transfer learning algorithms in a new R package glmtrans, which is available on CRAN.
|
Venue |
Click here to join the Zoom
Meeting Meeting ID: 9625852673
|
Chair |
Han Liu Jilin University
|
Hosts |
TEDS and Center for Quantitative Economics of Jilin University
|
|
A Modern Gauss-Markov Theorem, Econometrica, (2022), 90, 1283-1294.
Inference for Iterated GMM Under Misspecification, with Seojeong Lee, Econometrica, (2021), 89, 1419-14447.
Least Squares Model Averaging, Econometrica, (2007), 75, 1175-1189.
|
abc |
Title |
Standard Errors for Two-Way Clustering with Serially Correlated Time Effects
|
Abstract |
We propose improved standard errors and an asymptotic distribution theory for two-way clustered panels. Our proposed estimator and theory allow for arbitrary serial dependence in the common time effects, which is excluded by existing two-way methods, including the popular two-way cluster standard errors of Cameron, Gelbach, and Miller (2011) and the cluster bootstrap of Menzel (2021). Our asymptotic distribution theory is the first which allows for this level of inter-dependence among the observations. Under weak regularity conditions, we demonstrate that the least squares estimator is asymptotically normal, our proposed variance estimator is consistent, and t-ratios are asymptotically standard normal, permitting conventional inference. We present simulation evidence that confidence intervals constructed with our proposed standard errors obtain superior coverage performance relative to existing methods. We illustrate the relevance of the proposed method in an empirical application to a standard Fama-French three-factor regression.
|
|
|
|
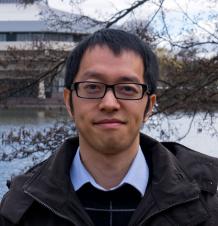 |
Date |
August/19/2022 |
Time |
Japan Standard Time (UTC+9h) |
|
16:00~17:30 |
Speaker |
Degui Li University of York
|
Title |
Estimating Time-Varying Networks for High-Dimensional Time Series
|
Abstract |
We explore time-varying networks for high-dimensional locally stationary time series, using large VAR with both transition and (error) precision matrices evolving smoothly over time. Two types of time-varying graphs are considered: one containing directed edges of Granger causality linkages, and the other containing undirected edges of partial correlation linkages. Under the sparse structural assumption, we propose a penalised local linear estimation method with weighted time-varying (or group) LASSO to jointly estimate the transition matrices and identify their significant entries, and a time-varying CLIME method to estimate the precision matrix. The estimated transition and precision matrices are then used to determine the time-varying network structures. Under some mild conditions, we derive the theoretical properties of the proposed estimates including the oracle and consistency properties. Extensive simulation studies are provided to illustrate the finite-sample performance.
|
Venue |
Click here to join the Zoom
Meeting Meeting ID: 846 1570 0936
Passcode: 403626
|
Chair |
Qu Feng Nanyang Technological University
|
Hosts |
TEDS and Nanyang Technological University
|
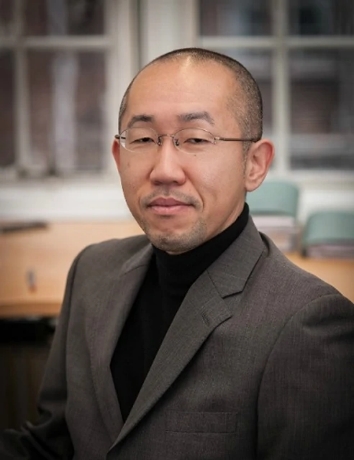 |
Date |
July/08/2022 |
Time |
Japan Standard Time (UTC+9h) |
|
11:30~13:00 |
Speaker |
Taisuke OtsuThe London School of Economics and Political Sciences
|
Title |
Likelihood Inference Under Alternative Asymptotics
|
Abstract |
The seminar will be based on the following three papers: (i) Empirical Likelihood for Network Data (with Yukitoshi Matsushita); (ii) Multiway Empirical Likelihood (with Harold Chiang and Yukitoshi Matsushita); (iii) Jackknife Empirical Likelihood: Small Bandwidths, Sparse Network and High-Dimensional Asymptotics (with Yukitoshi Matsushita).
|
Venue |
Click here to join the Zoom
Meeting Meeting ID: 313 176 9518
Passcode: 119549
|
Chair |
Qihui Chen The Chinese University of Hong Kong, Shenzhen
|
Hosts |
TEDS and The Chinese University of Hong Kong, Shenzhen
|
|
Date |
May/27/2022 |
Time |
Japan Standard Time (UTC+9h) |
|
9:30 ~ 11:00 |
Speaker |
Zhentao Shi Georgia Institute of Technology
|
Title |
Boosted Hodrick-Prescott Filter Is More General Than You Think |
Abstract |
Recent historical economic events such as the Great Recession and the Covid-19 recession have reignited the debate on econometric methodology for filtering low-frequency macroeconomic time series. While the Hodrick-Prescott (HP) filter remains, arguably, the off-the-shelf choice, the boosted HP (bHP) filter emerges as its modern machine learning upgrade for the data-rich environment. This paper sheds light on understanding the versatility of bHP in fitting time series of various nonstationary patterns. We establish a new asymptotic result that each iteration of the HP filter shrinks complex exponential functions proportionally toward zero. This founding opens a unified approach to tackle trends generated by unit root processes, higher order integrated processes, and local-to-unity processes. In an asymptotic framework where the penalty tuning parameter expands with the sample size, this theoretical property explains the smoothing effect of the original HP filter, and more importantly, it guarantees that the bHP filter consistently restores all the underlying trending processes mentioned above, in conjunction with potential deterministic trends and structural breaks. When it is applied to the universe of the FRED-QD dataset, bHP timely captures the downturns at crises and recessions and the recoveries that followed. Buttressed by its sound theoretical coverage and empirical performance, the automated bHP filtering procedure is well suited as a general-purpose machine for low-frequency macroeconomic time series.
|
Chair |
Xinyu Zhang Chinese Academy of Sciences
|
Hosts |
TEDS and Chinese Academy of Sciences
|
|
Date |
April/26/2022 |
Time |
Japan Standard Time (UTC+9h) |
|
10:50 ~ 12:20 |
Speaker |
Katsumi Shimotsu The University of Tokyo
|
Title |
Testing the Order of Multivariate Normal Mixture Models |
Abstract |
Finite mixtures of multivariate normal distributions have
been widely used in empirical applications in diverse fields such as statistical genetics and
statistical finance. Testing the number of components in multivariate normal mixture models is a
long-standing challenge even in the most important case of testing homogeneity. This paper develops
a likelihood-based test of the null hypothesis of M components against the alternative hypothesis of
M+1 components for a general M>=1. We derive the asymptotic distribution of the proposed EM test
statistic under the null hypothesis and local alternatives and show the validity of parametric
bootstrap. The simulations show that the proposed test has good finite sample size and power
properties.
|
Zoom |
Click here to join the Zoom
Meeting Meeting ID: 857 7302 7215 Passcode: 674245
|
Venue |
Koganei Campus, Hosei University. If you want to join in person, please contact qliu@hosei.ac.jp.
|
Chair |
Qingfeng Liu Hosei University
|
Hosts |
TEDS and Hosei University
|
|
Date |
April/12/2022 |
Time |
Japan Standard Time (UTC+9h) |
|
10:00 ~ 11:30 |
Speaker |
Jinchi Lv University of Southern California
|
Title |
High-Dimensional Knockoffs Inference for Time Series Data |
Abstract |
The recently introduced framework of model-X knockoffs provides a flexible tool for exact finite-sample false discovery rate (FDR) control in variable selection in arbitrary dimensions without assuming any dependence structure of the response on covariates. It also completely bypasses the use of conventional p-values, making it especially appealing in high-dimensional nonlinear models. Existing works have focused on the setting of independent and identically distributed observations. Yet time series data is prevalent in practical applications in various fields such as economics and social sciences. This motivates the study of model-X knockoffs inference for time series data. In this paper, we make some initial attempt to establish the theoretical and methodological foundation for the model-X knockoffs inference for time series data. We suggest the method of time series knockoffs inference (TSKI) by exploiting the idea of subsampling to alleviate the difficulty caused by the serial dependence. We establish sufficient conditions under which the original model-X knockoffs inference combined with subsampling still achieves the asymptotic FDR control. Our technical analysis reveals the exact effect of serial dependence on the FDR control. To alleviate the practical concern on the power loss because of reduced sample size cause by subsampling, we exploit the idea of knockoffs with copies and multiple knockoffs. Under fairly general time series model settings, we show that the FDR remains to be controlled asymptotically. To theoretically justify the power of TSKI, we further suggest the new knockoff statistic, the backward elimination ranking (BE) statistic, and show that it enjoys both the sure screening property and controlled FDR in the linear time series model setting. The theoretical results and appealing finite-sample performance of the suggested TSKI method coupled with the BE are illustrated with several simulation examples and an economic inflation forecasting application. This is a joint work with Chien-Ming Chi, Yingying Fan and Ching-Kang Ing.
|
Video |
Click here to watch the video
|
Chair |
Yoshimasa Uematsu Hitotsubashi University
|
Hosts |
TEDS and Hitotsubashi University
|
|
Date |
March/14/2022 |
Time |
Japan Standard Time (UTC+9h) |
|
15:00 ~ 16:30 |
Speaker |
Chu-An Liu Academia Sinica
|
Title |
Model Averaging Prediction by K-Fold Cross-Validation
|
Abstract |
This paper considers the model averaging prediction in a
quasi-likelihood framework that allows for parameter uncertainty and model misspecification. We
propose an averaging prediction that selects the data-driven weights by minimizing a K-fold
cross-validation. We provide two theoretical justifications for the proposed method. First, when
all candidate models are misspecified, we show that the proposed averaging prediction using
K-fold cross-validation weights is asymptotically optimal in the sense of achieving the lowest
possible prediction risk. Second, when the model set includes correctly specified models, we
demonstrate that the proposed K-fold crossvalidation asymptotically assigns all weights to the
correctly specified models. Monte Carlo simulations show that the proposed averaging prediction
achieves lower empirical risk than other existing model averaging methods. As an empirical
illustration, the proposed method is applied to credit card default prediction.
|
Video |
Click here to watch the video
(Passcode: P&pr4fRK)
|
Chair |
Nianqing (Paul) Liu Shanghai
University of Finance & Economics
|
Hosts |
TEDS and Otaru University of Commerce
|
|
Date |
Mar/03/2022 |
Time |
Japan Standard Time (UTC+9h) |
|
10:00 ~ 11:30 |
Speaker |
Yuan Liao Rutgers University
|
|
Yuan Liao is an Associate Professor of Economics at Rutgers
University. He received his Ph.D. in Statistics from Northwestern University in 2010. Before joining
Rutgers, Yuan held a position as Assistant Professor at University of Maryland, and worked at
Princeton University as a postdoc.
|
Title |
Inference for low rank estimation |
Abstract |
This paper studies the inferential theory for estimating
low-rank matrices. It also provides an inference method for the average treatment effect as an
application. We show that the least square estimation of eigenvectors following the nuclear norm
penalization attain the asymptotic normality. The key contribution of our method is that it does not
require sample splitting. In addition, this paper allows dependent observation patterns and
heterogenous observation probabilities. We illustrate the proposed method in simulation experiments
and the empirical study about the impact of the presidential vote on allocating the U.S. federal
budget to the states.
|
Video |
Click here to watch the video
|
Chair |
Qu Feng Nanyang
Technological University
|
Hosts |
TEDS and Nanyang Technological University
|
|
Date |
Feb/23/2022 |
Time |
Japan Standard Time (UTC+9h) |
|
15:00 ~ 16:30 |
Speaker |
Myoung-jae Lee Korea University
|
|
Professor Myoung-jae Lee is an econometrician and statistician at Korea
University. He received his Ph.D. in economics from University of Wisconsin-
Madison in 1989. Since then, he held regular positions in various universities
around the world, including Pennsylvania State University, Tilburg University,
Singapore Management University, Chinese University of Hong Kong,
and
Australian National University. He published more than 80 papers
on
economics, statistics, political science, sociology, transportation research, and
medical science. His papers appeared in many top-rated journals such as
Econometrica, Journal of the Royal Statistical Society (Series B), Biometrika,
Transportation Research (Part B), Po-litical Analysis, and Sociological Methods
& Research. Myoung-jae Lee also published five single-authored books from
Springer, Academic Press and Oxford University Press, induding Micro-
econometrics for policy, program, and treatment effects (2005), Micro.
Econometrics (2010), and Matching, regression discontinuity, difference in
differences, and beyond (2016).
|
Title |
OLS and IVE for Binary Treatment with
Propensity or
Instrument Score Residual
|
Abstract |
Given an endogenous/confounded binary treatment D, a response Y with its
potential versions
(YO, Y1) and covariates X, finding the treatment effect is difficult if Y is not continuous,
even
when a
binary instrumental variable (IV) Z is available. We show that, for any form of Y (continuous,
binary,
mixed,..), there exists a decomposition Y = u(X) + u1(X)D + error with E/error | Z,X) = 0, where
-1(X) =
E(Y1- Y0| complier, X) and 'compliers' are those who get treated if and only if Z = 1. First,
using
the
decomposition, instrumental variable estimator (IVE) is applicable with polynomial
approximations
for
MO(X) and u1(X) to obtain a linear model for Y. Second, better yet, an 'instrumental residual
estimator
(IRE)' with Z-E(Z|X) as an IV for D can be applied, and IRE is consistent for the
'E(Z|X)-overlap'
weighted
average of m1(X), which becomes E(Y1 - YO| complier) for randomized Z. Third, going further,
'weighted IRE' can be done which is consistent for E(u1(X)}. Empirical analyses as well as a
simulation
study are provided to illustrate our approaches.
|
Venue |
Click here to join the Zoom
Meeting Meeting ID: 955 0385 2198
Passcode: NTUECON
|
Chair |
Qu Feng Nanyang
Technological University
|
Hosts |
TEDS and Nanyang Technological University
|
|
Date |
Feb/18/2022 |
Time |
Japan Standard Time (UTC+9h) |
|
18:00 ~ 19:30 |
Speaker |
Takashi Yamagata University of York
|
|
Takashi Yamagata is Professor in Econometrics and the director of Centre for
Panel Data Analysis (PanDA) at the University of York, Specially Appointed
Professor at Osaka University, and Visiting Professor at Tohoku University. His
main research interests are in panel data econometrics methods, including
model estimation and inference for short and large panel data, and their
applications to economics and finance.
His work has been published in leading econometrics journals such as Journal
of Econometrics (8), Journal of Business and Economic Statistics
Econometrics Journal, and Econometric Reviews, among other outlets.
|
Title |
Discovering the Network Granger Causality in Large Vector
Autoregressive Models
|
Abstract |
In this paper we propose novel inferential procedures for
network Granger-causality in high-
dimensional vector autoregressive (VAR) models. These procedures are designed to control the
false
discovery rate (FDR) of each element in the coefficient matrices in the large VAR models. The
first
procedure is based on multiple testing using the limiting normal distributions of St$-statistics
constructed by the debased lasso estimator. The second procedure is based on bootstrapping. The
theoretical properties of the proposed procedures, including FDR control and power guarantees,
are
investigated. The finite sample evidence suggests that both procedures can successfully control
the FDR
while maintaining high power. The proposed method is applied to find network Granger-causality
in the
US financial market and in regional house prices in the UK.
|
Venue |
Click here to join the Zoom
Meeting Meeting ID: 865 2637 7715 Passcode: 878035
|
Chair |
Wenjie Wang Nanyang Technological University
|
Hosts |
TEDS and Nanyang Technological University
|
|
Date |
Feb/09/2022 |
Time |
Japan Standard Time (UTC+9h) |
|
18:30~20:00 |
Speaker |
Xiaohua Yu Georg-August-University Göttingen
|
|
Xiaohua Yu is Professor of Agricultural Economics in Developing and Transition Countries at the
Department of Agricultural Economics and Rural Development and the Courant Research Centre
“Poverty,
Equity and Growth” at the University of Göttingen, Germany. He studied in Renmin University of
China
and Kyoto University in Japan, and obtained his Ph.D. from Pennsylvania State University in the
U.S.
in 2009. Since 2009, he has been employed as a junior professor and then a full professor at the
University of Göttingen.
His research interests focus on agricultural development, nutrition change, economic and
environmental changes in developing and transition countries and contexts such as China, South
Asia,
Southeast Asia and African countries. He currently serves as the associate editor for
agricultural
economics, the official journal of International Association of Agricultural Economists (IAAE)
and
is an editorial board member in a few international journals.
|
Title |
Pattern Recognition and Unsupervised Machine Learning in
Agricultural Economics: Some Applications
|
Abstract |
TBA |
Video |
Click here to watch the video
(Passcode: 9ScSx$e2)
|
Chair |
Naoya Sueishi Kobe University
|
Hosts |
Jointly hosted by TEDS, Joint Research Program of KIER, Kyoto University
and Econometrics Seminar of KIER, Kyoto University.
|
|
Date |
Jan/27/2022 |
Time |
Japan Standard Time (UTC+9h) |
|
10:00 ~ 11:30 |
Speaker |
Eiji Kurozumi Hitotsubashi University
He received Ph.D. in Economics from Hitotsubashi University in March 2000.
After the post doctoral position of Japan Society for Promotion and Science, he started working
at
Hitotsubashi university in October 2000.
His research field is theoretical time series analysis.
Prof Kurozumi’s works have been published in leading econometrics journals such as Journal of
Econometrics, Econometric Theory, Econometrics Journal, Econometric Review, and Journal of Time
Series Analysis.
He has also received the JSS Ogawa Award and JSS Research Award from the Japan Statistical
Society,
and Distinguished Author Award from the Journal of Time Series Analysis.
|
Title |
On the asymptotic behavior of bubble date estimators
|
Abstract |
In this study, we
extend the three-regime bubble model of
Pang, Du, and Chong (2021) to allow the forth regime followed by the
unit root process after recovery. We provide the asymptotic and finite
sample justification of the consistency of the collapse date estimator
in the two-regime AR(1) model. The consistency allows us to split the
sample before and after the date of collapse and to consider the
estimation of the date of exuberation and date of recovery separately.
We have also found that the limiting behavior of the recovery date
varies depending on the extent of explosiveness and recovering.
|
Chair |
Qu Feng Nanyang Technological University
|
Hosts |
TEDS and Nanyang Technological University
|
Video |
Click here to watch the video
Meeting
|
|
Date |
Jan/18/2022 |
Time |
Japan Standard Time (UTC+9h) |
|
18:00 ~ 19:30 |
Speaker |
Thomas Kneib Georg-August-University Göttingen
|
Title |
Multivariate Conditional Transformation Models |
Abstract |
Regression
models
describing the joint distribution of multivariate
response variables conditional on covariate information have become an
important aspect of contemporary regression analysis. However, a
limitation of such models is that they often rely on rather simplistic
assumptions, e.g.~a constant dependence structure that is not allowed to
vary with the covariates or the restriction to linear dependence between
the responses only. We propose a general framework for multivariate
conditional transformation models that overcomes such limitations and
describes the full joint distribution in a tractable and interpretable
yet flexible way. Among the particular merits of the framework are that
it can be embedded into likelihood-based inference (including results on
asymptotic normality) and allows the dependence structure to vary with
the covariates. In addition, the framework scales well beyond bivariate
response situations.
|
Venue |
Click here to join the Zoom
Meeting
|
Hosts |
Jointly hosted by TEDS, Joint Research Program of KIER, Kyoto University
and Econometrics Seminar of KIER, Kyoto University.
|
Chair |
Qingfeng Liu
Otaru University of Commerce
|
Video |
Click here to see the video
|